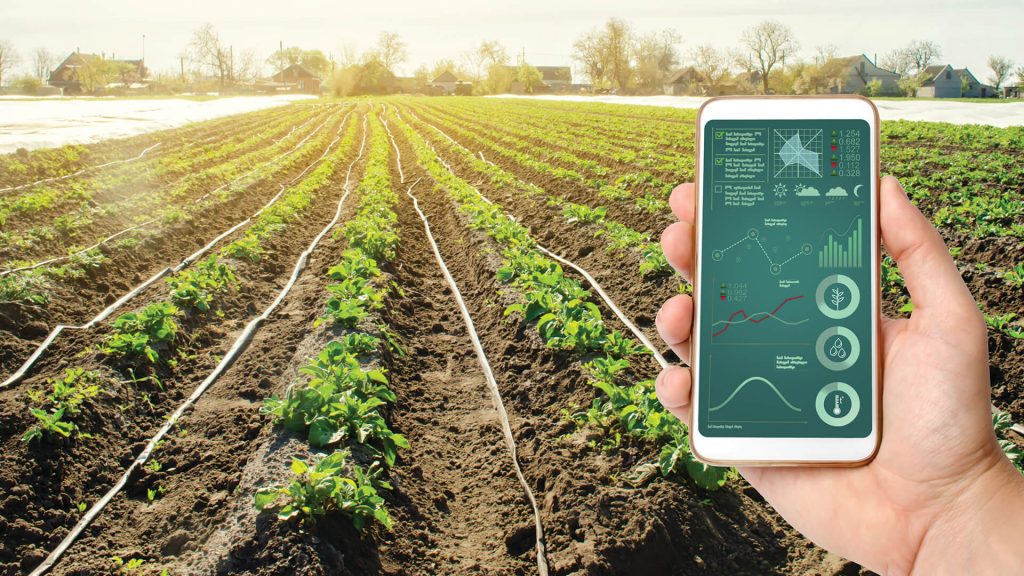
The future of Autonomous Farming starts with Vision
The challenges of the future
With a global population rapidly approaching 8 billion people, one of the ongoing challenges humanity faces is how to feed that many people in a way that is economical and sustainable. Agriculture has changed a lot over the past hundred years – modern methods of irrigation and land use has increased production dramatically, but with some unwanted side effects. These effects include things like depleted soil, nitrogen run-off into lakes and water systems and increased reliance on fossil fuels. It is clear that smarter use of land and resources moving into the future is both desirable and necessary. This is where technology – specifically autonomous farming and other new initiatives – can help.
What is autonomous farming?
While most modern farms use a wide variety of specialized mechanical equipment, that equipment still usually needs a significant amount of human operation and monitoring, and in the case of some kinds of crops, there are no viable existing alternatives to human harvesting by hand. Autonomous farming covers any type of farm equipment or innovation that reduces or removes the need for human operation or harvesting.
A simple example of an autonomous farming technology could be a dynamic irrigation system that uses sensors embedded in soil to monitor the level of moisture, and then waters plants accordingly. The key to any autonomous farming solution is quality information that allows for machines to take action without the need for the farmer to intervene. This information can help reduce wasted resources; if you’re monitoring the active level of moisture in the soil, you don’t need to continuously water just in case.
What kind of autonomous farming solutions could Intel® RealSense™ devices be used in?
Since Intel® RealSense™ Depth and tracking cameras have the ability to perceive their environments in detail and track their location within the space, one of the perfect uses for the cameras is autonomous tractors – with an Intel® RealSense™ tracking camera T265, the tractor could know where it is within a field, and the addition of one of the D400 series of depth cameras would allow for avoidance of unexpected obstacles such as people or animals. Multiple different pieces of equipment could also use the same localized map of the field, so that more than one machine could operate in the space at the same time, or successive, different types of autonomous farming robot could use that same map to identify specific plants or locations within the field for action.
With a D400 depth camera, additional capabilities are also unlocked – some fruits especially are hard to harvest mechanically due to location on the plant, or varying ripening times, or difficulty picking the fruit gently enough to not damage it.
Strawberries are a great example of a plant that is difficult to harvest mechanically. Strawberries grow under the leaves of their plants and ripen at different rates. They’re also very soft and delicate, making them difficult to pick with a robotic arm. During the harvesting process the plant and the fruit should both remain undamaged or bruised. A D400 depth camera added to an autonomous robot arm could serve to help solve all of these challenges. The robot could visit the field every day or few days during the peak harvest time, monitoring individual fruits on every plant. Each strawberry could be compared to an ideal strawberry – color, size and other attributes trained using machine learning techniques.
When each fruit is ready, the mechanical robot arm can be navigated around stems and leaves since the depth camera has collision avoidance capabilities. Since the size of the strawberry is easy to gauge with the depth camera, the robotic arm can be set to exactly the right size and pressure to pull the berry from the plant without damage. Such a system would potentially lead to less wasted or spoiled produce, increasing the yield. In recent years the lack of willing workers has also led to wasted produce, something a system like this isn’t vulnerable to.
Other problems
One of the issues with modern farming, as mentioned earlier, is soil depletion. Topsoil loses specific nutrients over time, especially if a field is used repeatedly for the same crop from year to year. This loss of nutrients means that many farmers need to use chemical fertilizers to replace them. Part of the reason farmers repeat the same crop from year to year is cost – if every different crop has different needs in terms of equipment, it’s not economically feasible to switch crops every year. Smarter autonomous farming robots could more easily adapt. Imagine being able to switch our strawberry picking robot earlier to one that could harvest zucchini, pull up weeds or harvest potato plants. Smarter equipment is more efficient in many different ways. Intel RealSense devices are designed to perceive and understand the world around them, enabling this kind of intelligent solution to age-old problems.
The future of farming
Today, various different companies and organizations are attempting to solve these and many more agricultural challenges – climate change, sustainability, resource depletion, water pollution, lack of viable land for farming, all of these are problems to be solved or have solutions which can be improved. Autonomous farms where farmers make decisions about what to do and how, but don’t have to sit on a tractor every day are still in our future. Some day soon though, everything you eat might be planted, tended to and harvested all by machines designed for the task.
Get started solving problems today!
Subscribe here to get blog and news updates.
You may also be interested in
“Intel RealSense acts as the eyes of the system, feeding real-world data to the AI brain that powers the MR
In a three-dimensional world, we still spend much of our time creating and consuming two-dimensional content. Most of the screens